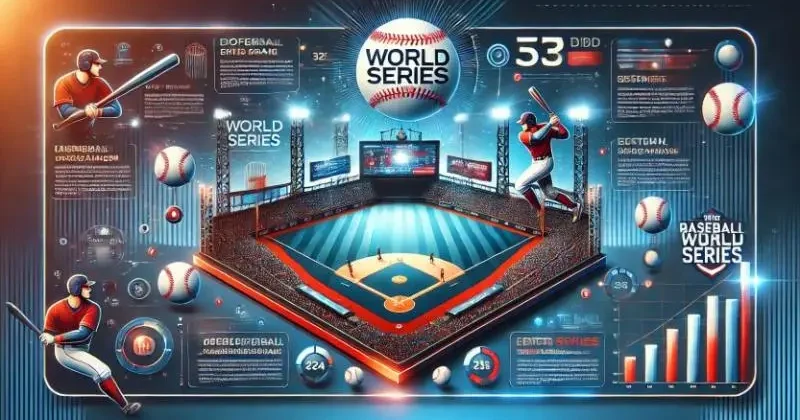
Share:
Baseball fans have long relied on basic statistics like batting averages and RBIs to evaluate players and teams. However, the game’s increasing complexity has led to the rise of advanced metrics that offer a deeper and more precise understanding of player performance. In this article, we’ll explore the evolving landscape of MLB baseball scores, shedding light on key metrics and offering insights into how they are changing the way fans and analysts view the game.
Going Beyond Traditional Stats
While runs and hits remain the core of a baseball game’s score, modern statistics have revolutionized how we interpret those numbers. The traditional batting average (BA), which simply measures a player’s hits divided by their at-bats, no longer offers the complete picture. New metrics such as exit velocity, launch angle, and expected weighted on-base average (xwOBA) are now seen as more valuable indicators of a player’s offensive contribution. These metrics are grounded in the fundamental laws of physics and advanced data analytics, and they provide predictive insights that traditional stats cannot.
For example, exit velocity measures the speed at which the ball leaves the bat, while launch angle refers to the angle at which the ball is hit. Together, these numbers offer a more precise understanding of a hitter’s ability to make quality contact, driving deeper analysis into a player’s potential for success.
Key Metrics Every Fan Should Track
With these new metrics in play, it’s important for fans to familiarize themselves with several key advanced statistics:
- xwOBA+: An evolution of the weighted on-base average (wOBA), xwOBA+ adjusts for factors like ballpark dimensions and league-wide trends. This metric helps gauge a player’s offensive value, factoring in the context of where and when the game is being played.
- Advanced Pitching Metrics: Metrics such as Fielding Independent Pitching (FIP) and Expected ERA (xERA) provide a more accurate picture of a pitcher’s effectiveness, excluding the influence of defense behind them. These advanced measures allow for a clearer understanding of a pitcher’s true skill.
- Sprinter Speed: A less conventional, yet increasingly significant metric, sprint speed measures how fast a player can run from one base to another. This has become a critical factor in assessing a player’s base-running ability and overall athleticism.
The Changing Landscape of Traditional Metrics
While new stats have garnered attention, traditional statistics like RBIs, ERA, and WHIP (walks plus hits per inning pitched) are not entirely obsolete. They are still essential, but their significance has shifted with the incorporation of context-driven advanced metrics.
For instance, RBIs (Runs Batted In) have become less valuable as a stand-alone stat. The strategic use of intentional walks, where pitchers purposely walk a batter to avoid a dangerous hitter, has diminished the correlation between RBIs and team success. Meanwhile, ERA and WHIP are still important, but they are increasingly supplemented by stats like xERA and xFIP, which attempt to factor in variables like defense and luck.
WAR (Wins Above Replacement) is another traditional stat that has evolved with advanced analytics. WAR compiles a player’s total contributions—batting, fielding, and baserunning—into a single number, allowing fans and analysts to compare players across different positions. This holistic approach helps teams make more informed decisions when evaluating talent.
Advanced Metrics in Action: A Deeper Dive
To truly understand the shift in baseball analysis, consider how Statcast has transformed player evaluation. Statcast uses high-speed cameras and radar to capture real-time data about everything from a player’s sprint speed to the exit velocity of their hits. It even tracks a player’s defensive range with precision, providing data on how many outs a player saves compared to his peers.
For example, a player with a modest batting average might impress with his sprint speed, turning routine singles into doubles or triples. Alternatively, a player’s exit velocity might indicate untapped power potential that traditional stats like batting average cannot reveal. By combining this data with traditional statistics like OPS (On-base Plus Slugging), teams can gain a more comprehensive understanding of player performance.
The Role of Machine Learning and Big Data
The intersection of big data and machine learning has created a new frontier for MLB analytics. Algorithms now analyze massive datasets to predict everything from game outcomes to player performance in specific situations. Machine learning can also predict a team’s likelihood of winning based on factors like pitch sequencing, player fatigue, and even social media sentiment about the game.
By analyzing millions of data points, these systems provide predictive insights that are incredibly accurate, offering real-time evaluations of team strategies and player performance. Such tools are invaluable for decision-makers looking to adjust their strategies on the fly and predict outcomes with greater precision.
Predicting Outcomes Using Advanced Metrics
Beyond player performance, modern analytics can also help fans and analysts predict game outcomes. A structured approach to prediction involves analyzing team batting averages, on-base percentages, slugging percentages, and ERA for pitchers. These metrics can be combined with advanced stats like wOBA and FIP to assess a team’s overall strength and potential.
For example, teams with high wOBA and strong FIP are likely to perform well, especially if they excel in areas like plate discipline and power hitting. Conversely, a team with low offensive metrics but strong pitching might struggle against high-scoring teams but perform better in low-scoring games. By applying regression analysis to these stats, one can predict the probabilities of team success with impressive accuracy.
The Future of MLB Analytics: Emerging Trends and Technologies
The future of MLB analytics promises even greater innovation. Wearable technologies and smart baseballs are set to revolutionize the way data is collected and analyzed. Sensors embedded in uniforms will capture detailed information on a player’s biomechanics, while AI-powered tools will process these data streams to provide instant, actionable insights.
This new wave of technology will not only improve game strategy but also enhance fan engagement by offering a more immersive experience. Real-time data integration could provide fans with deeper insights into every aspect of the game, from pitch sequencing to player fatigue.
Conclusion
As the landscape of MLB baseball scores evolves, so too does the way fans and analysts understand the game. Advanced metrics like xwOBA, launch angle, and exit velocity provide a more nuanced view of player performance, while machine learning and predictive modeling offer insights into game outcomes. By embracing these modern tools and metrics, fans can develop a deeper appreciation for the game’s complexity and anticipate the next wave of innovation in baseball analytics.